I am going to perform image classification with a ResNet50 deep learning model in this tutorial. I am using the CIFAR-10 dataset to train and test the model, code is written in Python. ResNet50 is a residual deep learning neural network model with 50 layers. ResNet was the winning model of the ImageNet (ILSVRC) 2015 competition and is a popular model for image classification, it is also often used as a backbone model for object detection in an image.
A neural network includes weights, a score function and a loss function. A neural network learns in a feedback loop, it adjusts its weights based on the results from the score function and the loss function. A simple neural network includes three layers, an input layer, a hidden layer and an output layer. Deep learning neural network models have more than 3 layers and convolutional layers is often used for image classification.
Deep neural network models is hard to train because of the vanishing gradient problem, repeated multiplication makes the gradient extremly small and causes the model to stop learning. ResNet solves the vanishing gradient problem with skip connection that allows input to flow in a shortcut to an activation layer.
Dataset and Libraries
I am using the CIFAR-10 dataset (download), it is a collection of 60 000 images in 10 different categories. Every image is stored in a folder with a name that corresponds to a category, 50 000 images is used for training and 10 000 images is used for testing/validation. The dataset is balanced as every category has the same number of images. It is a 10 % probabilty to classify an image correctly and this is our baseline performance, our model need to perform better than this. I am using the following libraries: os, random, numpy, pickle, cv2 and keras
.
Training
A ResNet50 model is created if it does not exist one on the disk already. A model is loaded if training has been performed before, to enable the model to continue its training (transfer learning). A ResNet50 model needs about 200 epochs of training to perform really well, I have only been able to train it in 25 epochs. The learning rate can be adjusted to speed up or slow down training time. The model and classes is saved to disk after each training session. Output from a training session is shown below the code.
# Import libraries
import os
import keras
import pickle
import numpy as np
# Get a ResNet50 model
def resnet50_model(classes=1000, *args, **kwargs):
# Load a model if we have saved one
if(os.path.isfile('C:\\DATA\\Python-data\\CIFAR-10\\models\\resnet_50.h5') == True):
return keras.models.load_model('C:\\DATA\\Python-data\\CIFAR-10\\models\\resnet_50.h5')
# Create an input layer
input = keras.layers.Input(shape=(None, None, 3))
# Create output layers
output = keras.layers.ZeroPadding2D(padding=3, name='padding_conv1')(input)
output = keras.layers.Conv2D(64, (7, 7), strides=(2, 2), use_bias=False, name='conv1')(output)
output = keras.layers.BatchNormalization(axis=3, epsilon=1e-5, name='bn_conv1')(output)
output = keras.layers.Activation('relu', name='conv1_relu')(output)
output = keras.layers.MaxPooling2D((3, 3), strides=(2, 2), padding='same', name='pool1')(output)
output = conv_block(output, 3, [64, 64, 256], stage=2, block='a', strides=(1, 1))
output = identity_block(output, 3, [64, 64, 256], stage=2, block='b')
output = identity_block(output, 3, [64, 64, 256], stage=2, block='c')
output = conv_block(output, 3, [128, 128, 512], stage=3, block='a')
output = identity_block(output, 3, [128, 128, 512], stage=3, block='b')
output = identity_block(output, 3, [128, 128, 512], stage=3, block='c')
output = identity_block(output, 3, [128, 128, 512], stage=3, block='d')
output = conv_block(output, 3, [256, 256, 1024], stage=4, block='a')
output = identity_block(output, 3, [256, 256, 1024], stage=4, block='b')
output = identity_block(output, 3, [256, 256, 1024], stage=4, block='c')
output = identity_block(output, 3, [256, 256, 1024], stage=4, block='d')
output = identity_block(output, 3, [256, 256, 1024], stage=4, block='e')
output = identity_block(output, 3, [256, 256, 1024], stage=4, block='f')
output = conv_block(output, 3, [512, 512, 2048], stage=5, block='a')
output = identity_block(output, 3, [512, 512, 2048], stage=5, block='b')
output = identity_block(output, 3, [512, 512, 2048], stage=5, block='c')
output = keras.layers.GlobalAveragePooling2D(name='pool5')(output)
output = keras.layers.Dense(classes, activation='softmax', name='fc1000')(output)
# Create a model from input layer and output layers
model = keras.models.Model(inputs=input, outputs=output, *args, **kwargs)
# Print model
print()
print(model.summary(), '\n')
# Compile the model
model.compile(loss='categorical_crossentropy', optimizer=keras.optimizers.adam(lr=0.01, clipnorm=0.001), metrics=['accuracy'])
# Return a model
return model
# Create an identity block
def identity_block(input, kernel_size, filters, stage, block):
# Variables
filters1, filters2, filters3 = filters
conv_name_base = 'res' + str(stage) + block + '_branch'
bn_name_base = 'bn' + str(stage) + block + '_branch'
# Create layers
output = keras.layers.Conv2D(filters1, (1, 1), kernel_initializer='he_normal', name=conv_name_base + '2a')(input)
output = keras.layers.BatchNormalization(axis=3, name=bn_name_base + '2a')(output)
output = keras.layers.Activation('relu')(output)
output = keras.layers.Conv2D(filters2, kernel_size, padding='same', kernel_initializer='he_normal', name=conv_name_base + '2b')(output)
output = keras.layers.BatchNormalization(axis=3, name=bn_name_base + '2b')(output)
output = keras.layers.Activation('relu')(output)
output = keras.layers.Conv2D(filters3, (1, 1), kernel_initializer='he_normal', name=conv_name_base + '2c')(output)
output = keras.layers.BatchNormalization(axis=3, name=bn_name_base + '2c')(output)
output = keras.layers.add([output, input])
output = keras.layers.Activation('relu')(output)
# Return a block
return output
# Create a convolution block
def conv_block(input, kernel_size, filters, stage, block, strides=(2, 2)):
# Variables
filters1, filters2, filters3 = filters
conv_name_base = 'res' + str(stage) + block + '_branch'
bn_name_base = 'bn' + str(stage) + block + '_branch'
# Create block layers
output = keras.layers.Conv2D(filters1, (1, 1), strides=strides, kernel_initializer='he_normal', name=conv_name_base + '2a')(input)
output = keras.layers.BatchNormalization(axis=3, name=bn_name_base + '2a')(output)
output = keras.layers.Activation('relu')(output)
output = keras.layers.Conv2D(filters2, kernel_size, padding='same', kernel_initializer='he_normal', name=conv_name_base + '2b')(output)
output = keras.layers.BatchNormalization(axis=3, name=bn_name_base + '2b')(output)
output = keras.layers.Activation('relu')(output)
output = keras.layers.Conv2D(filters3, (1, 1), kernel_initializer='he_normal', name=conv_name_base + '2c')(output)
output = keras.layers.BatchNormalization(axis=3, name=bn_name_base + '2c')(output)
shortcut = keras.layers.Conv2D(filters3, (1, 1), strides=strides, kernel_initializer='he_normal', name=conv_name_base + '1')(input)
shortcut = keras.layers.BatchNormalization(axis=3, name=bn_name_base + '1')(shortcut)
output = keras.layers.add([output, shortcut])
output = keras.layers.Activation('relu')(output)
# Return a block
return output
# Train a model
def train():
# Variables, 25 epochs so far
epochs = 1
batch_size = 32
train_samples = 10 * 5000 # 10 categories with 5000 images in each category
validation_samples = 10 * 1000 # 10 categories with 1000 images in each category
img_width, img_height = 32, 32
# Get the model (10 categories)
model = resnet50_model(10)
# Create a data generator for training
train_data_generator = keras.preprocessing.image.ImageDataGenerator(
rescale=1./255,
shear_range=0.2,
zoom_range=0.2,
horizontal_flip=True)
# Create a data generator for validation
validation_data_generator = keras.preprocessing.image.ImageDataGenerator(
rescale=1./255,
shear_range=0.2,
zoom_range=0.2,
horizontal_flip=True)
# Create a train generator
train_generator = train_data_generator.flow_from_directory(
'C:\\DATA\\Python-data\\CIFAR-10\\train',
target_size=(img_width, img_height),
batch_size=batch_size,
color_mode='rgb',
shuffle=True,
class_mode='categorical')
# Create a test generator
validation_generator = validation_data_generator.flow_from_directory(
'C:\\DATA\\Python-data\\CIFAR-10\\test',
target_size=(img_width, img_height),
batch_size=batch_size,
color_mode='rgb',
shuffle=True,
class_mode='categorical')
# Start training, fit the model
model.fit_generator(
train_generator,
steps_per_epoch=train_samples // batch_size,
validation_data=validation_generator,
validation_steps=validation_samples // batch_size,
epochs=epochs)
# Save model to disk
model.save('C:\\DATA\\Python-data\\CIFAR-10\\models\\resnet_50.h5')
print('Saved model to disk!')
# Get labels
labels = train_generator.class_indices
# Invert labels
classes = {}
for key, value in labels.items():
classes[value] = key.capitalize()
# Save classes to file
with open('C:\\DATA\\Python-data\\CIFAR-10\\classes.pkl', 'wb') as file:
pickle.dump(classes, file)
print('Saved classes to disk!')
# The main entry point for this module
def main():
# Train a model
train()
# Tell python to run main method
if __name__ == '__main__': main()
1510/1562 [============================>.] - ETA: 1:12 - loss: 0.5207 - accuracy: 0.8224
1511/1562 [============================>.] - ETA: 1:11 - loss: 0.5205 - accuracy: 0.8225
1512/1562 [============================>.] - ETA: 1:09 - loss: 0.5207 - accuracy: 0.8224
1513/1562 [============================>.] - ETA: 1:08 - loss: 0.5206 - accuracy: 0.8224
1514/1562 [============================>.] - ETA: 1:07 - loss: 0.5207 - accuracy: 0.8224
1515/1562 [============================>.] - ETA: 1:05 - loss: 0.5207 - accuracy: 0.8224
1516/1562 [============================>.] - ETA: 1:04 - loss: 0.5207 - accuracy: 0.8224
1517/1562 [============================>.] - ETA: 1:02 - loss: 0.5207 - accuracy: 0.8224
1518/1562 [============================>.] - ETA: 1:01 - loss: 0.5206 - accuracy: 0.8225
1519/1562 [============================>.] - ETA: 1:00 - loss: 0.5206 - accuracy: 0.8225
1520/1562 [============================>.] - ETA: 58s - loss: 0.5207 - accuracy: 0.8225
1521/1562 [============================>.] - ETA: 57s - loss: 0.5205 - accuracy: 0.8225
1522/1562 [============================>.] - ETA: 55s - loss: 0.5204 - accuracy: 0.8226
1523/1562 [============================>.] - ETA: 54s - loss: 0.5206 - accuracy: 0.8225
1524/1562 [============================>.] - ETA: 53s - loss: 0.5206 - accuracy: 0.8224
1525/1562 [============================>.] - ETA: 51s - loss: 0.5204 - accuracy: 0.8225
1526/1562 [============================>.] - ETA: 50s - loss: 0.5204 - accuracy: 0.8225
1527/1562 [============================>.] - ETA: 48s - loss: 0.5204 - accuracy: 0.8225
1528/1562 [============================>.] - ETA: 47s - loss: 0.5205 - accuracy: 0.8225
1529/1562 [============================>.] - ETA: 46s - loss: 0.5206 - accuracy: 0.8225
1530/1562 [============================>.] - ETA: 44s - loss: 0.5209 - accuracy: 0.8224
1531/1562 [============================>.] - ETA: 43s - loss: 0.5208 - accuracy: 0.8225
1532/1562 [============================>.] - ETA: 41s - loss: 0.5207 - accuracy: 0.8225
1533/1562 [============================>.] - ETA: 40s - loss: 0.5208 - accuracy: 0.8224
1534/1562 [============================>.] - ETA: 39s - loss: 0.5208 - accuracy: 0.8224
1535/1562 [============================>.] - ETA: 37s - loss: 0.5208 - accuracy: 0.8224
1536/1562 [============================>.] - ETA: 36s - loss: 0.5209 - accuracy: 0.8224
1537/1562 [============================>.] - ETA: 34s - loss: 0.5211 - accuracy: 0.8224
1538/1562 [============================>.] - ETA: 33s - loss: 0.5212 - accuracy: 0.8223
1539/1562 [============================>.] - ETA: 32s - loss: 0.5214 - accuracy: 0.8222
1540/1562 [============================>.] - ETA: 30s - loss: 0.5212 - accuracy: 0.8223
1541/1562 [============================>.] - ETA: 29s - loss: 0.5212 - accuracy: 0.8223
1542/1562 [============================>.] - ETA: 27s - loss: 0.5215 - accuracy: 0.8222
1543/1562 [============================>.] - ETA: 26s - loss: 0.5215 - accuracy: 0.8222
1544/1562 [============================>.] - ETA: 25s - loss: 0.5214 - accuracy: 0.8222
1545/1562 [============================>.] - ETA: 23s - loss: 0.5215 - accuracy: 0.8222
1546/1562 [============================>.] - ETA: 22s - loss: 0.5217 - accuracy: 0.8222
1547/1562 [============================>.] - ETA: 20s - loss: 0.5217 - accuracy: 0.8221
1548/1562 [============================>.] - ETA: 19s - loss: 0.5216 - accuracy: 0.8222
1549/1562 [============================>.] - ETA: 18s - loss: 0.5214 - accuracy: 0.8222
1550/1562 [============================>.] - ETA: 16s - loss: 0.5216 - accuracy: 0.8221
1551/1562 [============================>.] - ETA: 15s - loss: 0.5217 - accuracy: 0.8221
1552/1562 [============================>.] - ETA: 13s - loss: 0.5216 - accuracy: 0.8221
1553/1562 [============================>.] - ETA: 12s - loss: 0.5219 - accuracy: 0.8221
1554/1562 [============================>.] - ETA: 11s - loss: 0.5221 - accuracy: 0.8221
1555/1562 [============================>.] - ETA: 9s - loss: 0.5220 - accuracy: 0.8221
1556/1562 [============================>.] - ETA: 8s - loss: 0.5219 - accuracy: 0.8221
1557/1562 [============================>.] - ETA: 6s - loss: 0.5220 - accuracy: 0.8221
1558/1562 [============================>.] - ETA: 5s - loss: 0.5221 - accuracy: 0.8220
1559/1562 [============================>.] - ETA: 4s - loss: 0.5222 - accuracy: 0.8219
1560/1562 [============================>.] - ETA: 2s - loss: 0.5225 - accuracy: 0.8218
1561/1562 [============================>.] - ETA: 1s - loss: 0.5225 - accuracy: 0.8217
1562/1562 [==============================] - 2205s 1s/step - loss: 0.5224 - accuracy: 0.8218 - val_loss: 1.1075 - val_accuracy: 0.7412
Saved model to disk!
Saved classes to disk!
Evaluation
CIFAR-10 images has low resoultion, every image have a size of 32×32 pixels. The model has only been trained for 25 epochs, with a learning rate of 0.01. The model and classes is loaded from disk before evaluation. A category is selected at random and images in the category is shuffled at random. Output from an evaluation is shown below the code.
# Import libraries
import os
import cv2
import keras
import random
import pickle
import numpy as np
# Evaluate the model
def evaluate():
# Load the model
model = keras.models.load_model('C:\\DATA\\Python-data\\CIFAR-10\\models\\resnet_50.h5')
# Load classes
classes = {}
with open('C:\\DATA\\Python-data\\CIFAR-10\\classes.pkl', 'rb') as file:
classes = pickle.load(file)
# Get a list of categories
categories = os.listdir('C:\\DATA\\Python-data\\CIFAR-10\\test')
# Get a category a random
category = random.choice(categories)
# Print the category
print(category)
# Get images in a category
images = os.listdir('C:\\DATA\\Python-data\\CIFAR-10\\test\\' + category)
# Randomize images to get different images each time
random.shuffle(images)
# Loop images
blocks = []
for i, name in enumerate(images):
# Limit the evaluation
if i > 6:
break;
# Print the name
print(name)
# Get the image
image = cv2.imread('C:\\DATA\\Python-data\\CIFAR-10\\test\\' + category + '\\' + name)
# Get input reshaped and rescaled
input = np.array(image).reshape((1, 32, 32, 3)).astype('float32')/255
# Get predictions
predictions = model.predict(input).ravel()
# Print predictions
print(predictions)
# Get the class with the highest probability
prediction = np.argmax(predictions)
# Check if the prediction is correct
correct = True if classes[prediction].lower() == category else False
# Draw the image and show the best prediction
image = cv2.resize(image,(256,256))
cv2.putText(image, '{0}: {1} %'.format(classes[prediction], str(round(predictions[prediction] * 100, 2))), (12, 22), cv2.FONT_HERSHEY_DUPLEX, 0.7, (0, 0, 0), 2)
cv2.putText(image, '{0}: {1} %'.format(classes[prediction], str(round(predictions[prediction] * 100, 2))), (10, 20), cv2.FONT_HERSHEY_DUPLEX, 0.7, (65,105,225), 2)
cv2.putText(image, '{0}'.format('CORRECT!' if correct else 'WRONG!'), (12, 50), cv2.FONT_HERSHEY_DUPLEX, 0.7, (0, 0, 0), 2)
cv2.putText(image, '{0}'.format('CORRECT!' if correct else 'WRONG!'), (10, 48), cv2.FONT_HERSHEY_DUPLEX, 0.7, (0, 255, 0) if correct else (0, 0, 255), 2)
# Append the image
blocks.append(image)
# Display images and predictions
row1 = np.concatenate(blocks[0:3], axis=1)
row2 = np.concatenate(blocks[3:6], axis=1)
#cv2.imshow('Predictions', np.concatenate((row1, row2), axis=0))
cv2.imwrite('C:\\DATA\\Python-data\\CIFAR-10\\plots\\predictions.jpg', np.concatenate((row1, row2), axis=0))
cv2.waitKey(0)
# The main entry point for this module
def main():
# Evaluate the model
evaluate()
# Tell python to run main method
if __name__ == '__main__': main()
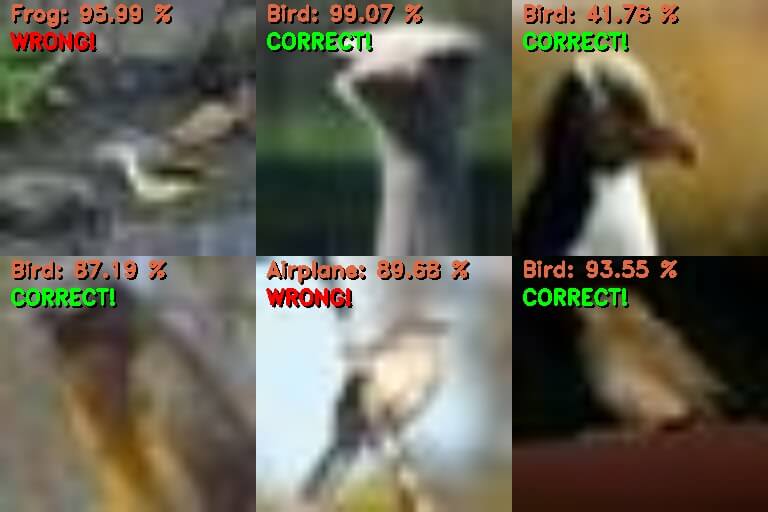
hello, do you have a repo with this code? i didn’t find the resnet50.h5 file to run this code and i need it to a studient project.
thanks for all, emanuel.
You need to run a training session before the resnet50.h5 file is created.
This sample is great, much appreciate.
when i try to evaluate the code it shows an error like
“ValueError: cannot reshape array of size 1 into shape (1,32,32,3)”
what does it mean “array of size 1”
Thanks for the article …
I get the following error
raise RuntimeError (‘You must compile your model before’
RuntimeError: You must compile your model before training / testing. Use `model.compile (optimizer, loss)`.
Maybe you can help me understand why?
Thank you
This method will compile the model: def resnet50_model(classes=1000, *args, **kwargs), make sure that you have correct paths to your file system. Paths in this example is correct on my file system.
Pingback: What is image classification in Python? - What Type Degree